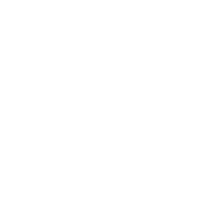
浏览全部资源
扫码关注微信
1.重庆科技大学 石油与天然气工程学院,重庆 401331
2.中国石油大庆油田有限责任公司第三采油厂,黑龙江 大庆 163000
3.青岛能源设计研究院有限公司,山东 青岛 266000
4.重庆科技大学 非常规油气开发研究院,重庆 401331
5.中国石油大学(华东) 储运与建筑工程学院,山东 青岛 266580
Published:25 December 2024,
Received:25 January 2024,
Revised:29 March 2024,
移动端阅览
张威,张伟文,李欣洋等.超重力反应器天然气脱碳过程反应传质智能预测[J].低碳化学与化工,2024,49(12):96-102.
ZHANG Wei,ZHANG Weiwen,LI Xinyang,et al.Intelligent prediction of mass transfer during natural gas decarburization in high gravity reactor[J].Low-carbon Chemistry and Chemical Engineering,2024,49(12):96-102.
张威,张伟文,李欣洋等.超重力反应器天然气脱碳过程反应传质智能预测[J].低碳化学与化工,2024,49(12):96-102. DOI: 10.12434/j.issn.2097-2547.20240032.
ZHANG Wei,ZHANG Weiwen,LI Xinyang,et al.Intelligent prediction of mass transfer during natural gas decarburization in high gravity reactor[J].Low-carbon Chemistry and Chemical Engineering,2024,49(12):96-102. DOI: 10.12434/j.issn.2097-2547.20240032.
超重力反应器具有优异的传质性能,在天然气脱碳领域具有广阔的应用前景。为了有效预测超重力反应器的天然气脱碳性能,首先搭建了一套超重力反应器天然气脱碳实验系统,考察了超重力因子、吸收液喷淋密度、混合气体积流量和进气组成等运行参数对模拟天然气脱碳效果的影响;然后采用无量纲方法建立了运行参数与传质系数之间的映射关系;最后基于最小二乘支持向量机(LSSVM)算法,建立了智能预测模型。结果表明,超重力因子和吸收液喷淋密度均存在最优值,分别为57.62和2.04 m
3
/(m
2
·h)。增大混合气体积流量,CO
2
脱除效果下降,但传质系数增大。在最优模型参数([
γ
t
d
]
= [13557.2021
9.5876
4
]
)下,智能预测模型预测结果的决定系数(
R
2
)和平均相对误差(
MRE
)分别为0.9519和0.0949,预测结果的相对误差在±20%以内,说明智能预测模型具有较高的准确性。
The high-gravity reactor has excellent mass transfer performance and has broad application prospects in the field of natural gas decarbonization. In order to effectively predict the natural gas decarburization performance of the high-gravity reactor
a set of natural gas decarburization experimental system based on high-gravity reactor was built
and the influences of operating parameters (high gravity factor
spray density o
f absorbent
mixture volume flow rate and intake composition
etc) on the CO
2
removal performance were investigated. Then a dimensionless method was adopted to establish the mapping relationship between operating parameters and mass transfer coefficient. Finally
based on the least square support vector machine (LSSVM) algorithm
the intelligent prediction model was established. The results show that both the high gravity factor and absorbent spray density have optimal values
which are 57.62 and 2.04 m
3
/(m
2
·h)
respectively. In addition
it is found that the CO
2
removal effect decreases while the mass transfer coefficient increases with the increases of mixture volume flow rate. Under the condition of optimal model parameter ([
γ
t
d
]
= [13557.2021
9.5876
4
]
)
the determination coefficient (
R
2
) and average relative error (
MRE
) of prediction results of intelligent prediction model are 0.9519 and 0.0949
respectively
and the relative error of prediction results is within ±20%. It demonstrates that the intelligent prediction model has high accuracy.
超重力反应器天然气脱碳LSSVM算法传质智能预测模型
high gravity reactornatural gas decarburizationLSSVM algorithmmass transferintelligent prediction model
周淑慧, 王军, 梁严. 碳中和背景下中国“十四五”天然气行业发展[J]. 天然气工业, 2021, 41(2): 171-182.
ZHOU S H, WANG J, LIANG Y. Development of China’s natural gas industry during the 14th Five-year Plan in the background of carbon neutrality [J]. Natural Gas Industry, 2021, 41(2): 171-182.
李孥, 王建良, 刘睿, 等. 碳中和目标下天然气产业发展的多情景构想[J]. 天然气工业, 2021, 41(2): 183-192.
LI N, WANG J L, LIU R, et al. Multi-scenario conception on the development of natural gas industry under the goal of carbon neutrality [J]. Natural Gas Industry, 2021, 41(2): 183-192.
洪宗平, 叶楚梅, 吴洪, 等. 天然气脱碳技术研究进展[J]. 化工学报, 2021, 72(12): 6030-6048.
HONG Z P, YE C M, WU H, et al. Research progress in CO2 removal technology of natural gas [J]. CIESC Journal, 2021, 72(12): 6030-6048.
花亦怀, 郭清, 冯颉, 等. 天然气脱碳单一胺液及混合胺液解吸性能研究[J]. 天然气化工—C1化学与化工, 2014, 39(1): 47-51.
HUA Y H, GUO Q, FENG S, et al. Study on desorption properties of single amine and mixed amines in natural gas decarbonization process [J]. Natural Gas Chemical Industry, 2014, 39(1): 47-51.
熊波, 陈健, 李克兵, 等. 工业排放气二氧化碳捕集与利用技术进展[J]. 低碳化学与化工, 2023, 48(1): 9-18.
XIONG B, CHEN J, LI K B, et al. Technical progress in carbon dioxide capture and utilization of industrial vent gas [J]. Low-Carbon Chemistry and Chemical Engineering, 2023, 48(1): 9-18.
蔡勇, 朱瑞松, 魏弢, 等. 二氧化碳捕集技术研究进展及其在驱油中的应用[J]. 低碳化学与化工, 2024, 49(1): 85-93.
CAI Y, ZHU R S, WEI T, et al. Research progress of carbon dioxide capture technologies and their application in enhanced oil recovery [J]. Low-Carbon Chemistry and Chemical Engineering, 2024, 49(1): 85-93.
ZHANG W, XIE P, LI Y X, et al. Hydrodynamic characteristics and mass transfer performance of rotating packed bed for CO2 removal by chemical absorption: A review [J]. Journal of Natural Gas Science and Engineering, 2020, 79: 103373.
邹海魁, 初广文, 赵宏, 等. 面向环境应用的超重力反应器强化技术: 从理论到工业化[J]. 中国科学: 化学, 2014, 44(9): 1413-1422.
ZOU H K, CHU G W, ZHAO H, et al. Process intensification of high-gravity reactor for environmental engineering: From fundamental to industrialization [J]. SCIENTIA SINICA (Chimica), 2014, 44(9): 1413-1422.
王云飞, 秦蕊, 郑利军, 等. 旋转填充床CFD模拟研究进展[J]. 化工进展, 2023, 42(S1): 1-9.
WANG Y F, QIN R, ZHENG L J, et al. Research progress of rotating packed bed simulation through CFD method [J]. Chemical Industry and Engineering Progress, 2023, 42(S1): 1-9.
JIAO W Z, LIU Y Z, QI G S, et al. Micromixing efficiency of viscous media in novel impinging stream-rotating packed bed reactor [J]. Industrial & Engineering Chemistry Research, 2012, 51: 7113-7118.
SAHA D. Prediction of mass transfer coefficient in rotating bed contactor (Higee) using artificial neural network [J]. Heat and Mass Transfer 2009, 45: 451-457.
LI W W, WU X L, JIAO W Z, et al. Modelling of dust removal in rotating packed bed using artificial neural networks (ANN) [J]. Applied Thermal Engineering, 2017, 112: 208-213.
LIU Z W, LIANG F N, LIU Y Z. Artificial neural network modeling of biosorption process using agricultural wastes in a rotating packed bed [J]. Applied Thermal Engineering, 2018, 140: 95-101.
常爱英, 吴铁军, 包鑫, 等. 基于最小二乘支持向量机的动力配煤着火特性预测模型[J]. 煤炭学报, 2010, 35(8): 1380-1383.
CAI A Y, WU T J, BAO X, et al. Ignition characteristic prediction model for blending coal based on least squares support vector machine [J]. Journal of China Coal Society, 2010, 35(8): 1380-1383.
董梅英, 祁贵生, 刘有智, 等. 错流旋转填料床传质特性影响因素的实验研究[J]. 过程工程学报, 2015, 15(6): 929-934.
DONG M Y, QI G S, LIU Y Z, et al. Experimental study on influential factors of mass transfer in a cross-flow rotating packed bed [J]. The Chinese Journal of Process Engineering, 2015, 15(6): 929-934.
WANG Y S, DONG Y N, ZHANG L L, et al. Carbon dioxide capture by non-aqueous blend in rotating packed bed reactor: Absorption and desorption investigation [J]. Separation and Purification Technology, 2021, 269: 118714.
ZHANG W, XIE P, LI Y X, et al. A machine learning model for predicting the mass transfer performance of rotating packed beds based on a least squares support vector machine approach [J]. Chemical Engineering Processing-Process Intensification, 2021, 165: 108432.
0
Views
0
下载量
0
CNKI被引量
Publicity Resources
Related Articles
Related Author
Related Institution